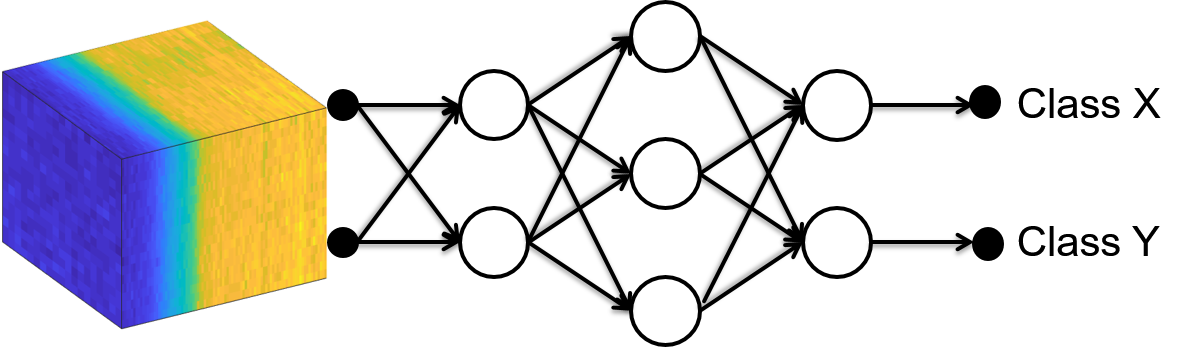
In the field of image processing, artificial neural networks are becoming increasingly important. Although the underlying concept has existed for a long time, they have been very successful, especially in recent years. A major contribution to this has been made by the increased computing power, which allows networks consisting of many layers (deep learning) to be trained. Convolutional Neural Networks (CNNs), which process data using a convolutional kernel, have achieved good results in the field of image processing. CNNs often also have pooling layers in which less relevant information is discarded.
Hyperspectral images, which have a high spectral resolution, have their origins in remote sensing. However, they are also increasingly used in industrial processes, such as defect detection.
The aim of this research is to investigate how hyperspectral images can be processed using deep learning methods. Due to the high number of color channels, problems arise that do not occur with color images with three channels. On the one hand, larger convolutional kernels and thus more parameters are required, on the other hand, there is little labelled data available for hyperspectral images. This leads to the danger that during training the parameters are too much adapted to the training data (overfitting) and thus bad results with new data are achieved. Therefore, methods are investigated which perform well with little labelled data and still learn suitable features. Such methods often belong to the area of unsupervised learning.